
Gonzalo Martínez-Alés Awarded New Suicide Research Grant
“Effectiveness of Pharmacological Interventions to Prevent Suicidal Behaviors Among Individuals at High Suicide Risk: A Target Trial Emulation” (Grant ECR-1-101-23) aims to apply innovative target trial emulation design using big data from EMR to investigate the relationship of medications with suicide risk.
CAUSALab Director Miguel Hernán will serve as a mentor alongside M. Mercedes Perez-Rodriguez from Mount Sinai Health System and Enrique Baca-Garcia from Universidad Autónoma de Madrid.

New Research Featured by CNN: Metformin & Pregnancy Risk
The study by Yu-Han Chiu et al published in Annals of Internal Medicine found that women with diabetes type 2 who continued using metformin as a treatment in their first trimester of pregnancy showed no increased risk for giving birth to a baby with major birth defects when compared with women who discontinued metformin.
Dr. Yu-Han Chiu, the lead author of the study, is a CAUSALab researcher collaborator and current faculty member at Penn State College of Medicine. This work was supported and advised by CAUSALab Director Miguel Hernán and Sonia Hernández-Díaz, Professor of Epidemiology and Co-Director of Harvard Program on Perinatal and Pediatric Pharmacoepidemiology (H4P) at Harvard T.H. Chan School of Public Health.
Read the full CNN article, “Use of popular diabetes drug in preconception or early pregnancy may not be tied to higher birth defect risk, studies suggest.”
Read the original publications in Annals of Internal Medicine:
-Metformin Use in the First Trimester of Pregnancy and Risk for Nonlive Birth and Congenital Malformations: Emulating a Target Trial Using Real-World Data
-Paternal Use of Metformin During the Sperm Development Period Preceding Conception and Risk for Major Congenital Malformations in Newborns

Pygformula for Causal Inference Published on CAUSALab GitHub
The pygformula package implements the non-iterative conditional expectation (NICE) algorithm of the g-formula algorithm. The g-formula can estimate an outcome’s counterfactual mean or risk under hypothetical treatment strategies (interventions) when there is sufficient information on time-varying treatments and confounders.
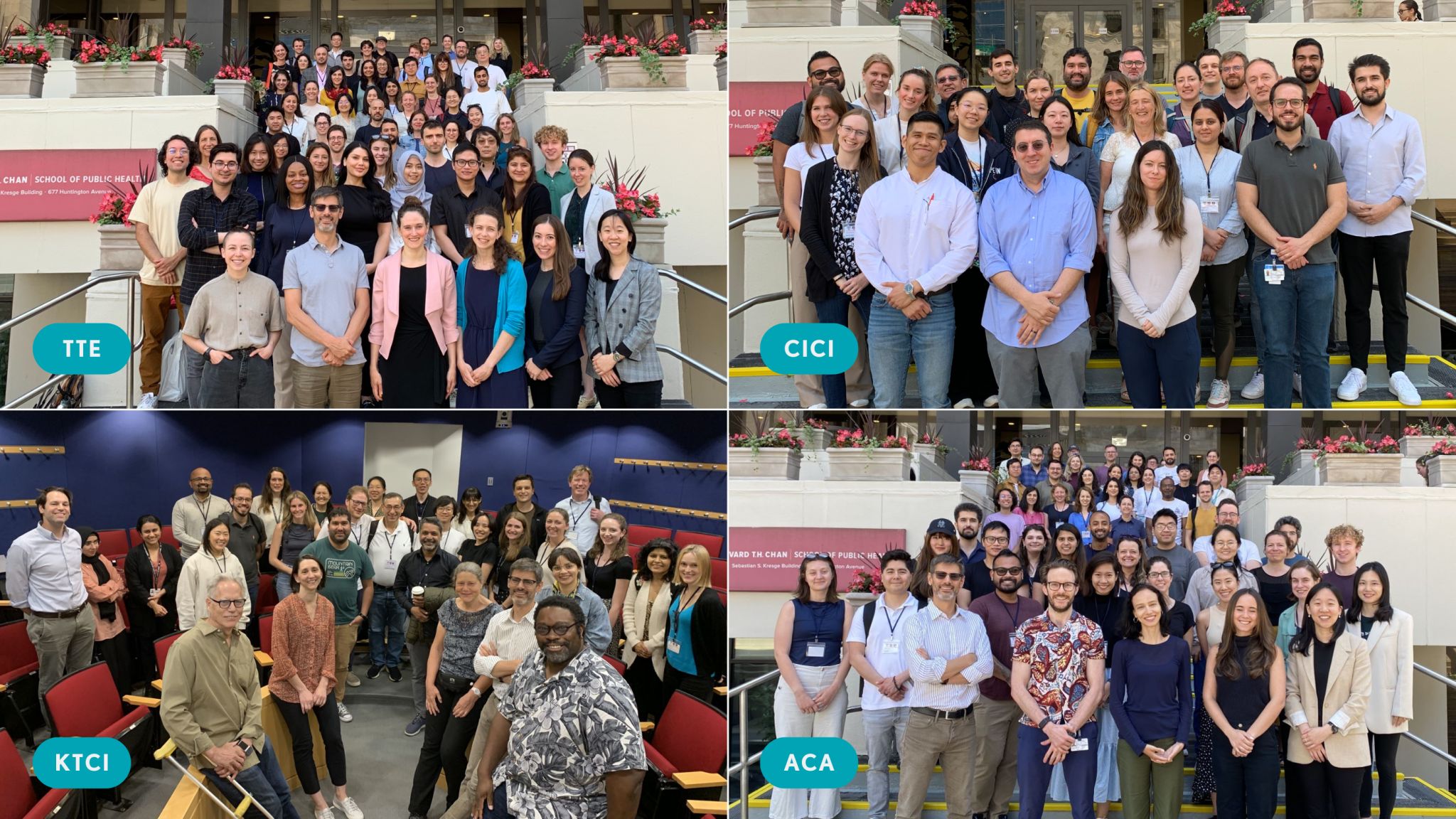
2024 Summer Courses on Causal Inference a Success
Attendees participated in four causal inference courses: Key Topics in Causal Inference (KTCI), Advanced Confounding Adjustment (ACA), Combining Information for Causal Inference (CICI), and Target Trial Emulation (TTE). Thank you to our wonderful instructors and fellows for their work this year!
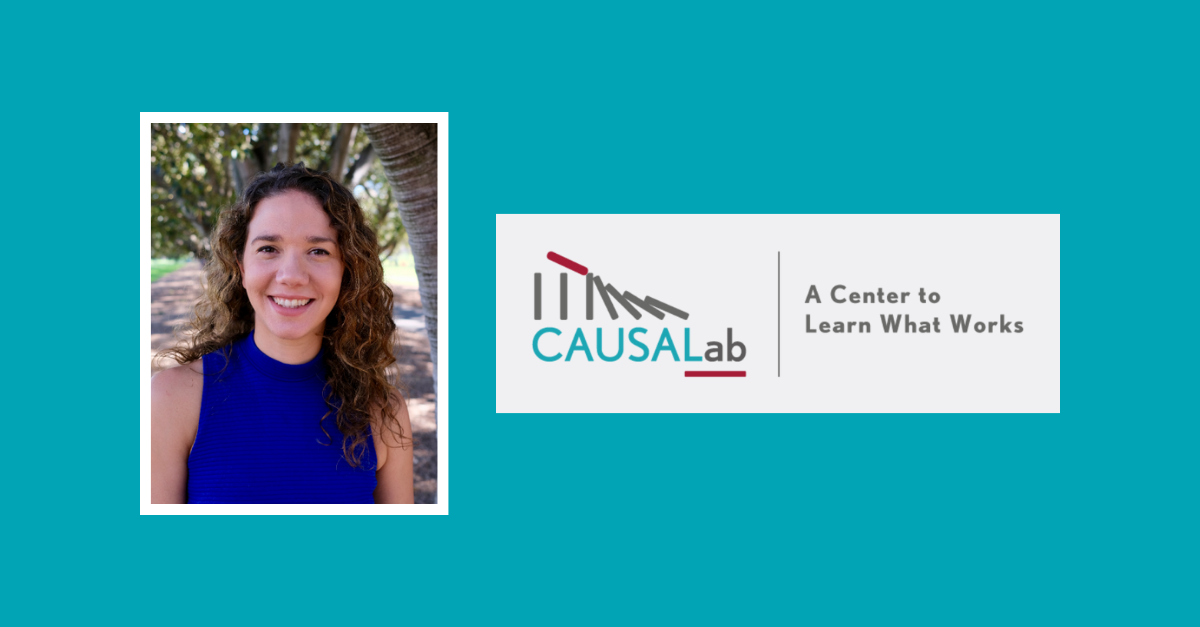
Daniela van Santen - Promotion
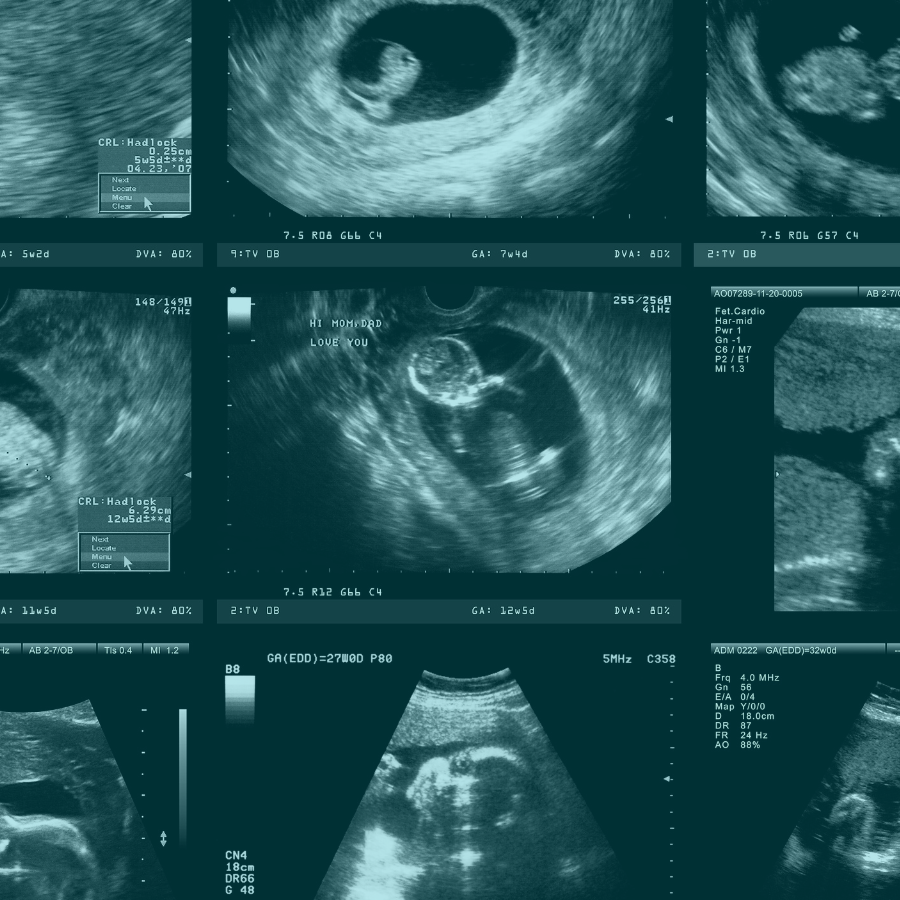
New Publication: Risk of Autism after Prenatal Topiramate, Valproate, or Lamotrigine Exposure
In a recent article co-authored by CAUSALab faculty member Sonia Hernández-Díaz and published in NEJM Group’s New England Journal of Medicine, researchers investigated this association using a pregnant population-based cohort. Children exposed to antiseizure medications during the second half of pregnancy (beginning gestational week 19) were compared to those not exposed in utero.
Findings suggest that topiramate —an antiseizure medication prescribed to treat epilepsy as well as migraines and bipolar disorder—does not appear to increase the risk of autism spectrum disorder among children exposed to it prenatally. While children exposed to all the studied antiseizure medications had a higher risk of autism than the general population, after adjusting for indication and other confounding variables, exposure to topiramate and lamotrigine was no longer associated, while prenatal exposure to valproate remained associated with additional risk.
This research was funded by the National Institute of Mental Health (NIMH). Read the full article
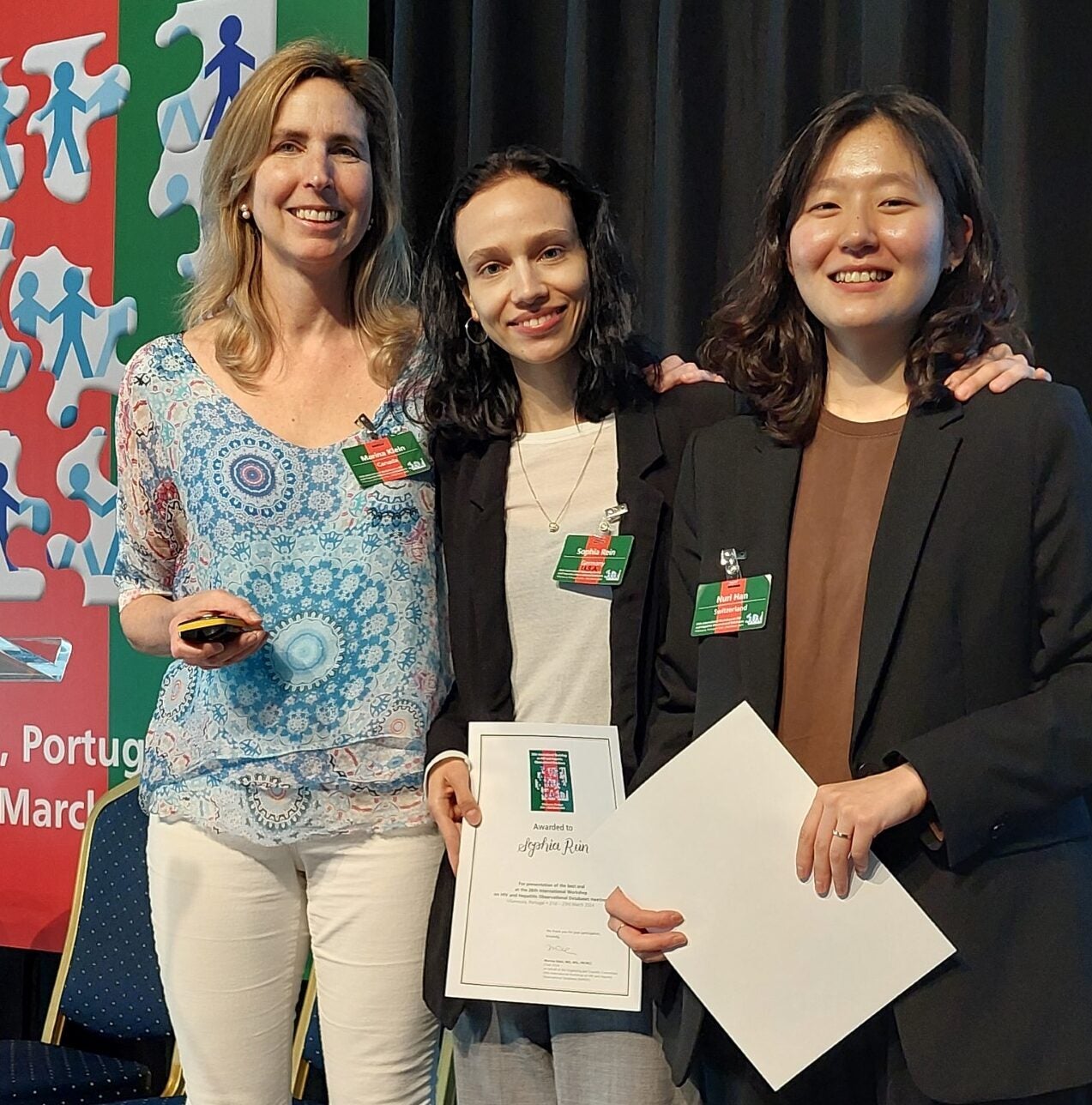
Sophia Rein Wins IWHOD 2024 Young Investigator Award
IWHOD brings HIV and hepatitis database researchers together to advance observational data analysis.
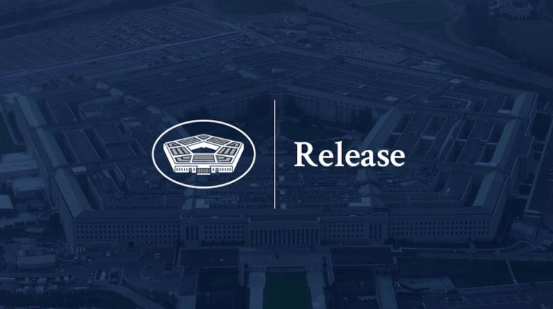
CAUSALab Announces Collaboration with Broad Institute of MIT and Harvard
The MURI program, founded in 1985, fosters cross-institution collaboration amongst teams with diverse expertise to accelerate scientific advancement on topics key to national defense.
US Department of Defense press release published March 8, 2024.
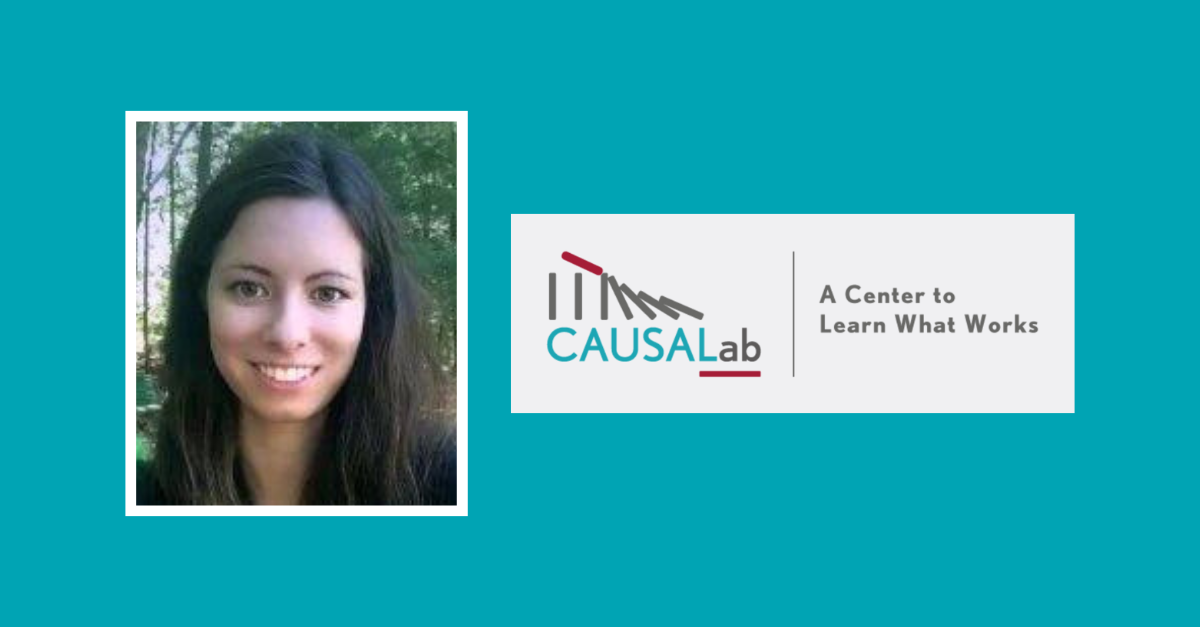
Sarah Robertson - Promotion
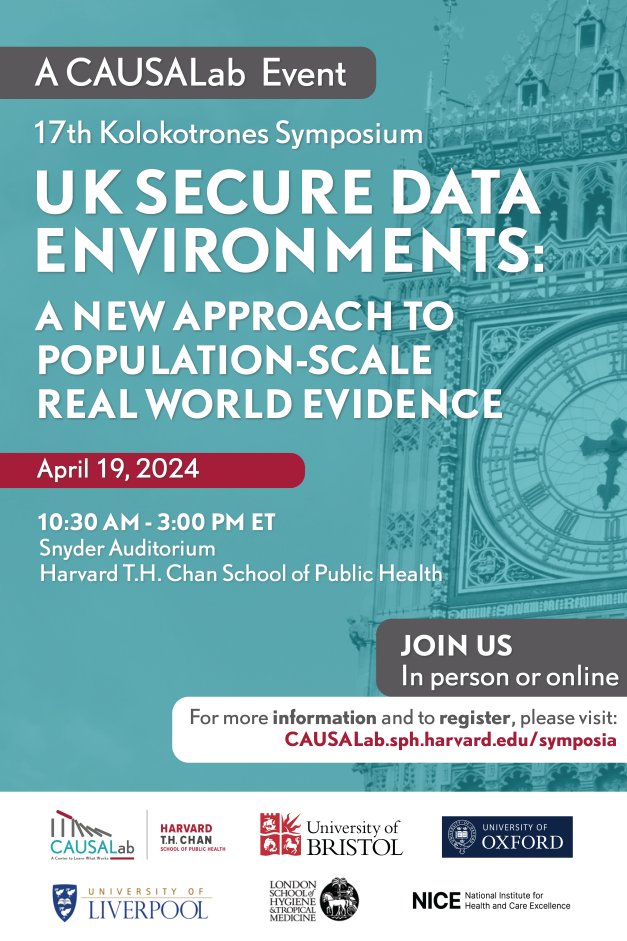
17th Kolokotrones Symposium Announced
Information about “UK Secure Data Environments: A New Approach to Population-Scale Real World Evidence” is live on the Symposium section of our website.
Register now on Eventbrite.
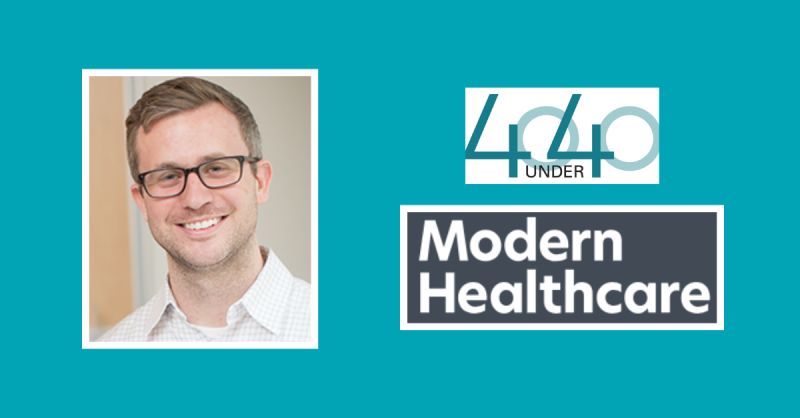
Andrew Beam Named Modern Healthcare 40 Under 40 Recipient
Andrew’s contributions to AI research & machine learning, both inside and outside the classroom, landed him a spot amongst this year’s class. His research in the neonatal intensive care setting is highlighted as a notable contribution to the field in his award. Andrew was nominated by NEJM AI, a monthly journal from NEJM Group on which he serves as Deputy Editor. The 40 Under 40 list is selected by senior editors at Modern Healthcare and features individuals who have demonstrated “perseverance, flexibility and innovation” while creating a measurable impact to any sector of healthcare.
Read the feature here.
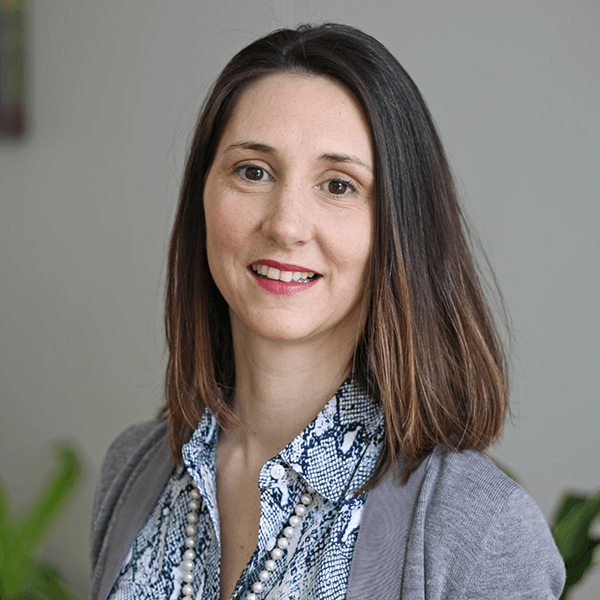
Sara Lodi Joins Summer Course Instructor Team
She joins Miguel Hernán, Judith Lok, James Robins, Eric Tchetgen Tchetgen & Tyler VanderWeele for the June 3-7, 2024 course.
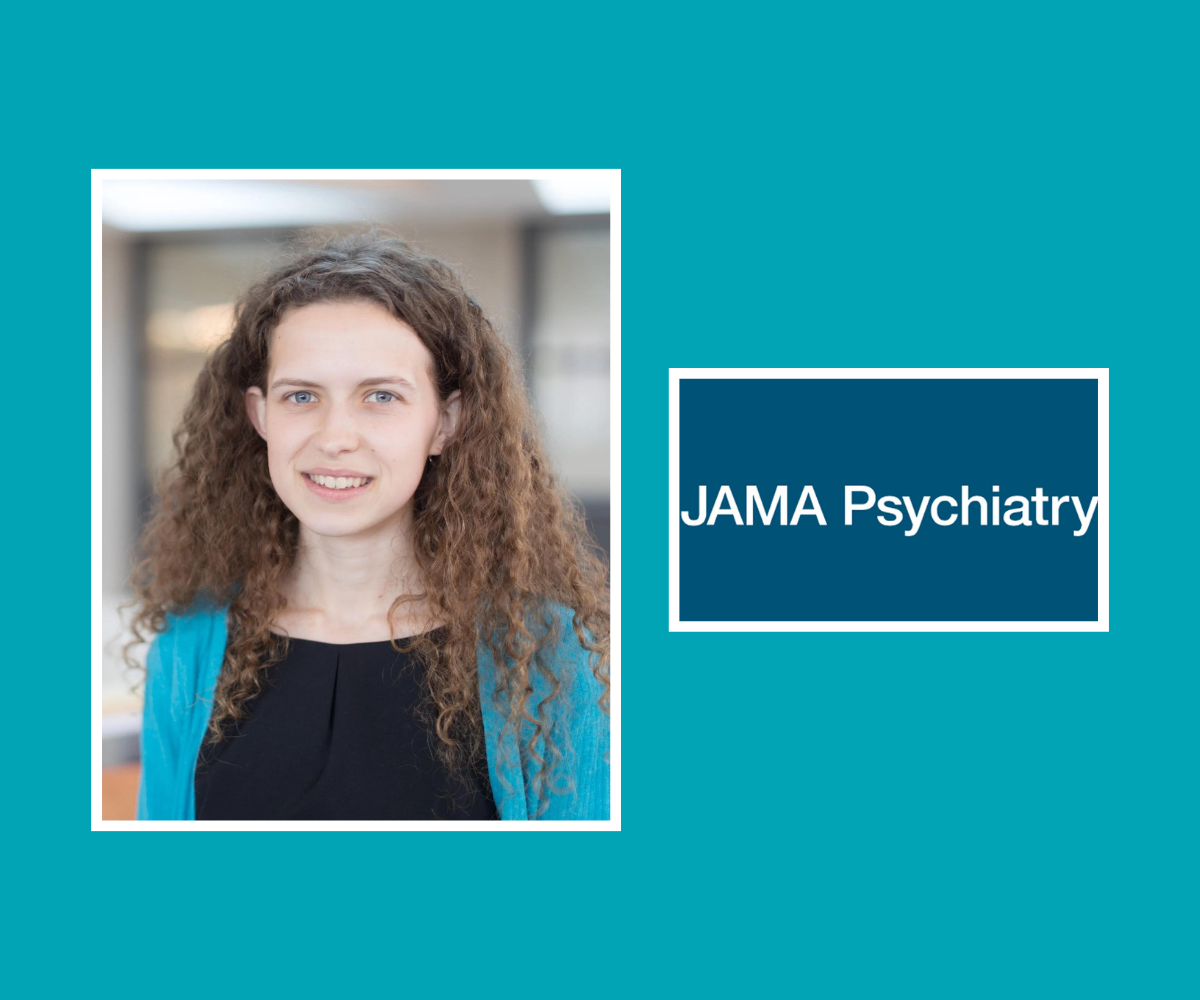
Elizabeth Diemer - JAMA Psychiatry Appointment
Check out her profile and recent publications, including “Falsification of the instrumental variable conditions in Mendelian randomization studies in the UK Biobank” published in European Journal of Epidemiology here.
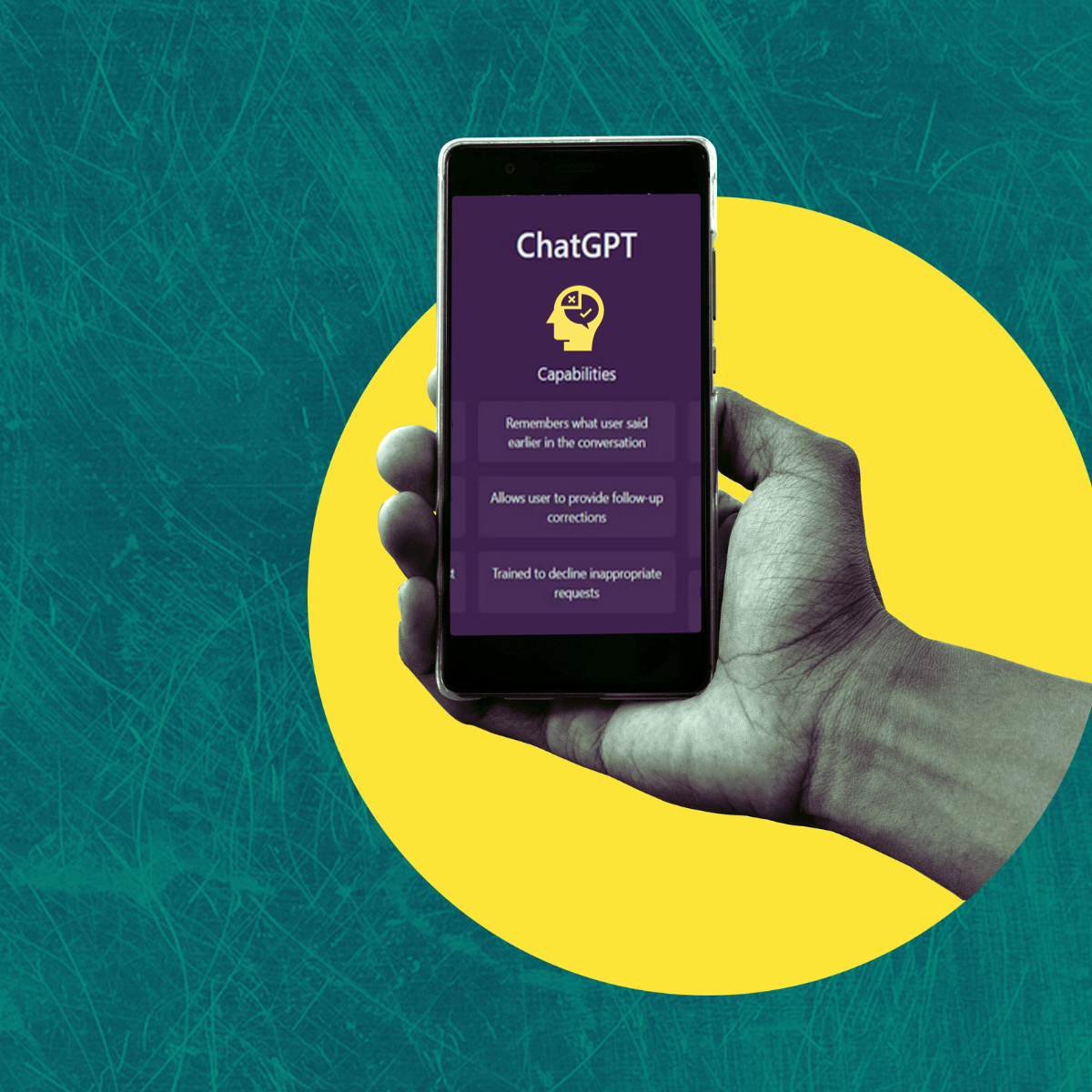
New Publication: Affiliation Bias in Peer Review of Abstracts by a Large Language Model
In a recent research letter published December 2023 and co-authored by CAUSALab researcher Denys Shay in addition to Dario von Wedel, Rico André Schmitt, Moritz Thiele, Raphael Leuner, Simone Redaelli and Maximilian S. Schaefer, researchers investigated this question by prompting ChatGPT-3.5 to act as a peer reviewer for 30 abstracts. They then tested affiliation combinations and analyzed corresponding acceptance & rejection rates. Analysis indicates an association between high-tier institutions and LLM acceptance rates may exist, but limitations must be taken into consideration.
Read the full letter published is JAMA here.
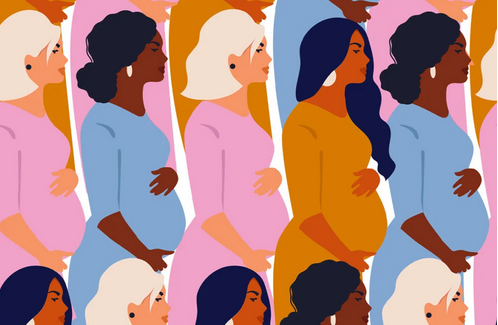
Article Feature: 16th Kolokotrones Symposium
Read the article here.
Watch the event recordings here.
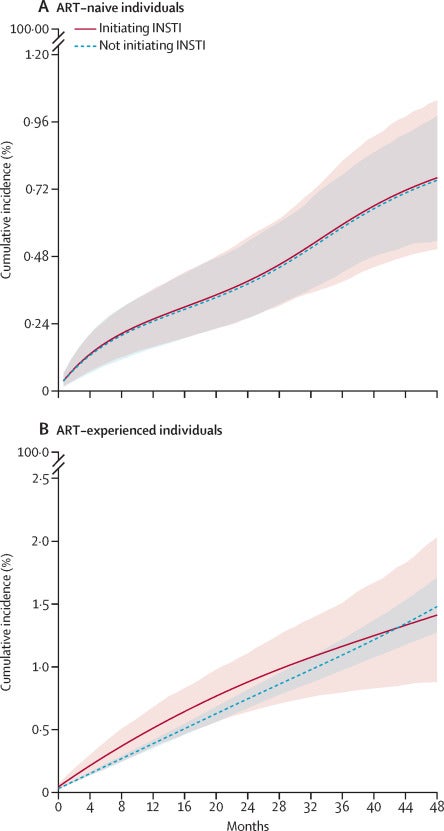
New Study: Integrase strand-transfer inhibitor use and cardiovascular events in adults with HIV: an emulation of target trials in the HIV-CAUSAL Collaboration and the Antiretroviral Therapy Cohort Collaboration
A recent observational study suggested that the risk of cardiovascular events could be higher among antiretroviral therapy (ART)-naive individuals with HIV who receive integrase strand-transfer inhibitor (INSTI)-based ART than among those who receive other ART regimens. The team aimed to emulate target trials separately in ART-naive and ART-experienced individuals with HIV to examine the effect of using INSTI-based regimens versus other ART regimens on the 4-year risk of cardiovascular events.
You can read the abstract and paper here.
Sophia was invited to a podcast from The Lancet HIV. Listen to her talk about the team’s research on Spotify here or on The Lancet HIV‘s website here.
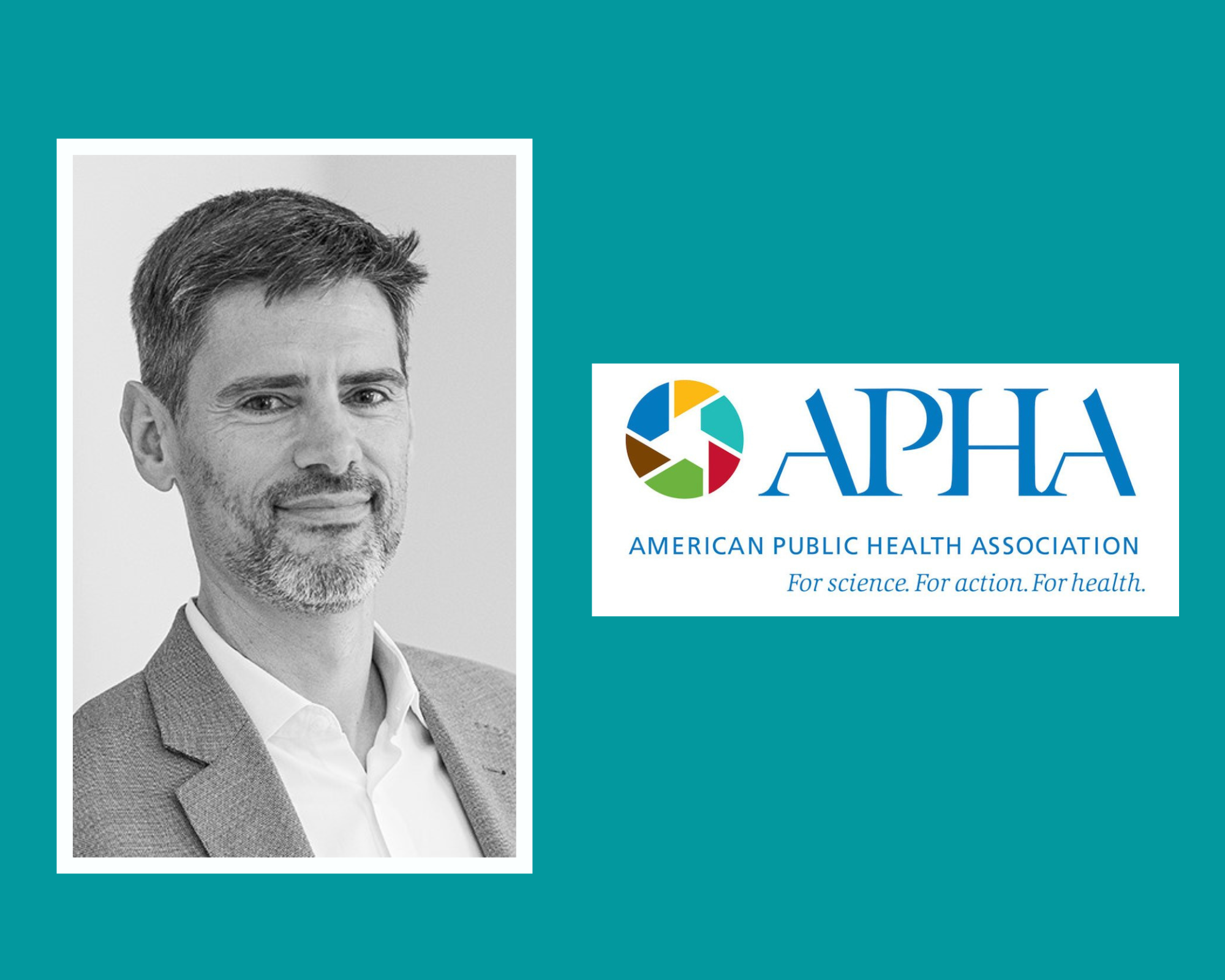
Miguel Hernan - Awarded 2023 Lowell Reed Lectureship Award
Dr. Hernan gave his lecture on “Making decisions is hard, but making decisions without data is even harder: Lessons for the next public health crisis” at the award ceremony of APHA’s Applied Public Health Statistics Section, at the APHA conference in Atlanta, GA on Tuesday, November 14, 2023.
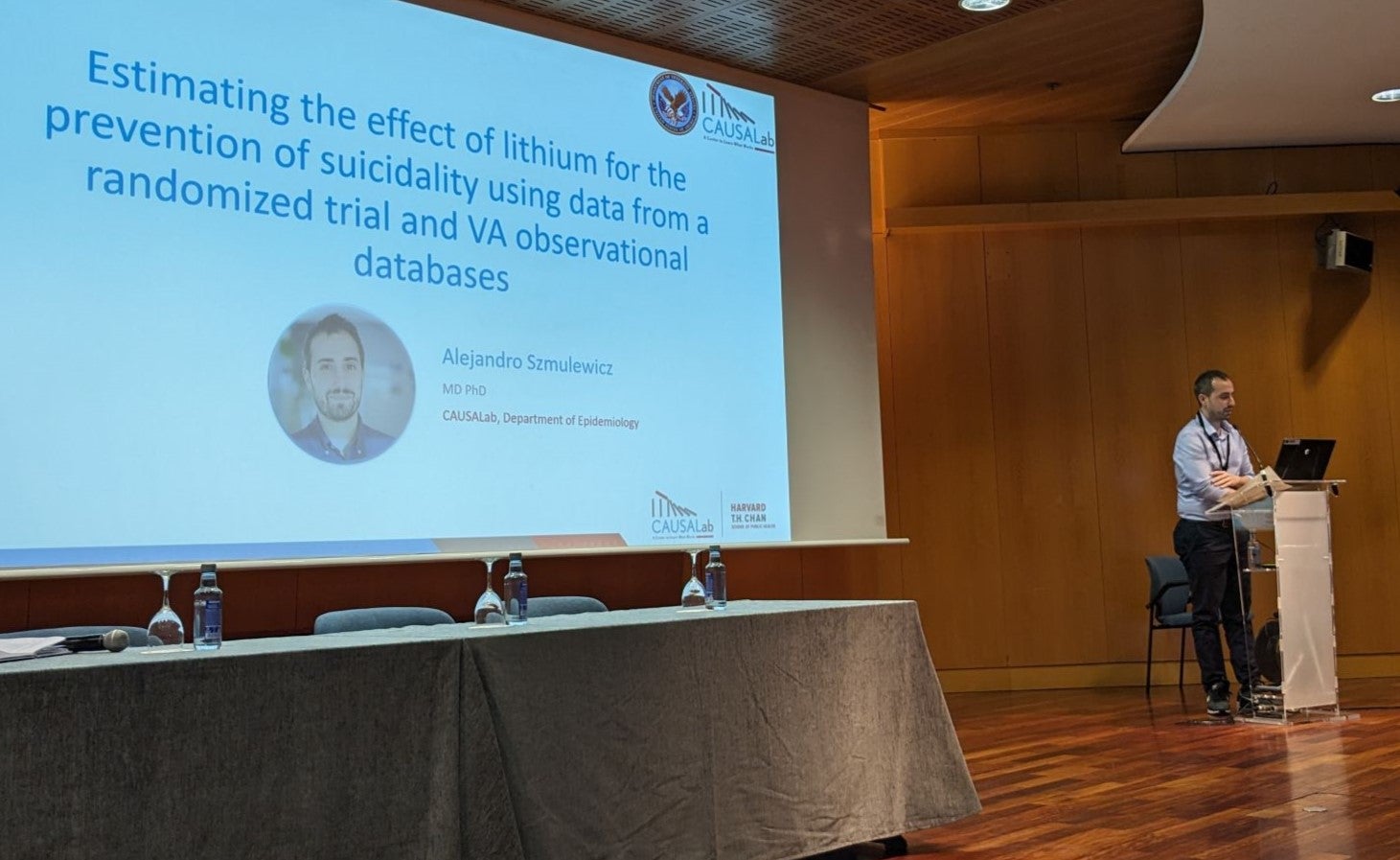
Alejandro Szmulewicz - International Summit on Suicide Research
Alejandro was also a featured panelist during the conference. His work is supported by VA Boston and the U.S. Department of Veteran Affairs.
The International Summit on Suicide Research engages an interdisciplinary group of researchers studying topics ranging from brain function, genetics, rates of suicide and factors that contribute to suicide as well as approaches to intervention and prevention.
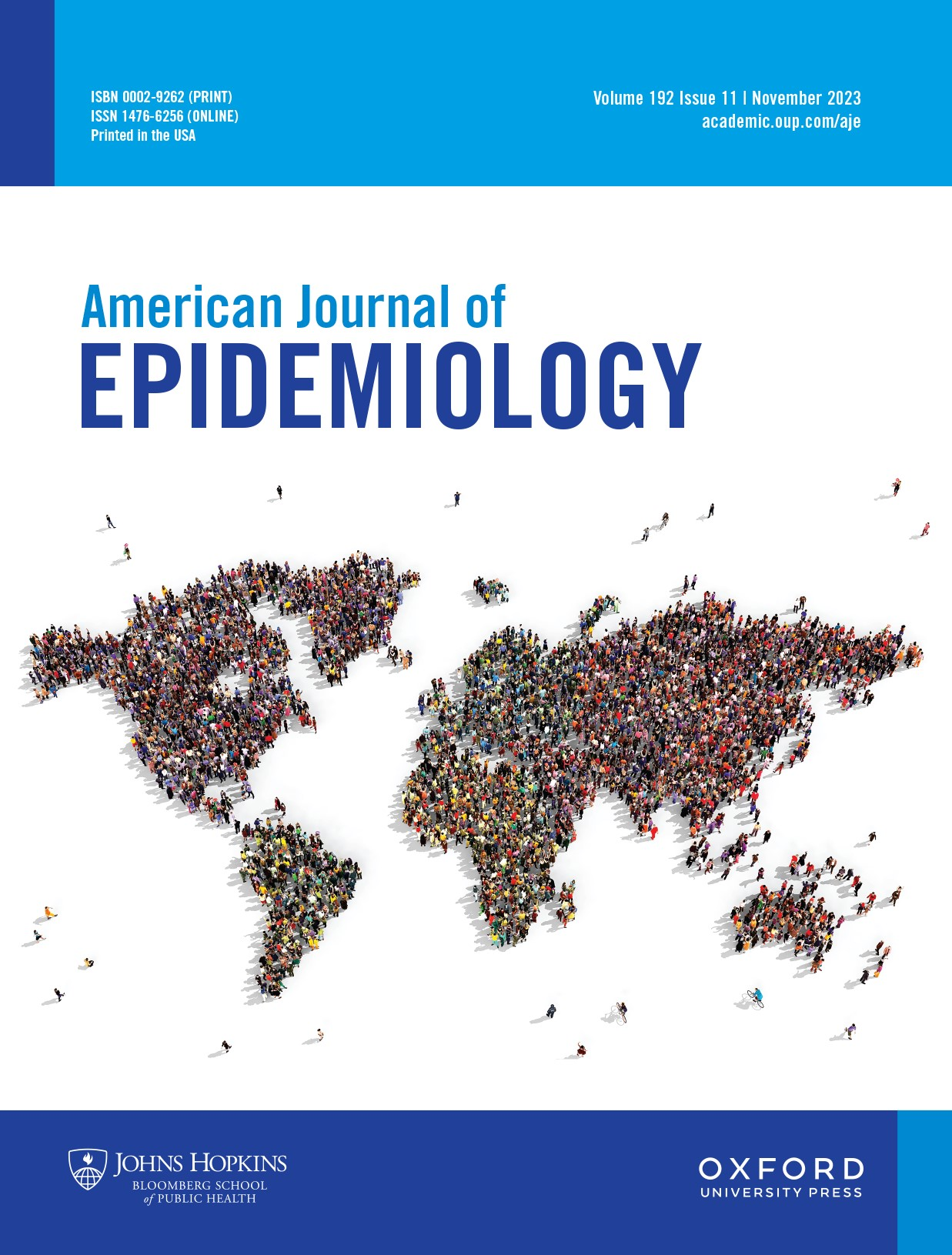
New Study: Evaluating Model Specification When Using the Parametric G-Formula in the Presence of Censoring
Abstract is shared below and you can access the paper here:
The noniterative conditional expectation (NICE) parametric g-formula can be used to estimate the causal effect of sustained treatment strategies. In addition to identifiability conditions, the validity of the NICE parametric g-formula generally requires the correct specification of models for time-varying outcomes, treatments, and confounders at each follow-up time point. An informal approach for evaluating model specification is to compare the observed distributions of the outcome, treatments, and confounders with their parametric g-formula estimates under the “natural course.” In the presence of loss to follow-up, however, the observed and natural-course risks can differ even if the identifiability conditions of the parametric g-formula hold and there is no model misspecification. Here, we describe 2 approaches for evaluating model specification when using the parametric g-formula in the presence of censoring: 1) comparing factual risks estimated by the g-formula with nonparametric Kaplan-Meier estimates and 2) comparing natural-course risks estimated by inverse probability weighting with those estimated by the g-formula. We also describe how to correctly compute natural-course estimates of time-varying covariate means when using a computationally efficient g-formula algorithm. We evaluate the proposed methods via simulation and implement them to estimate the effects of dietary interventions in 2 cohort studies.
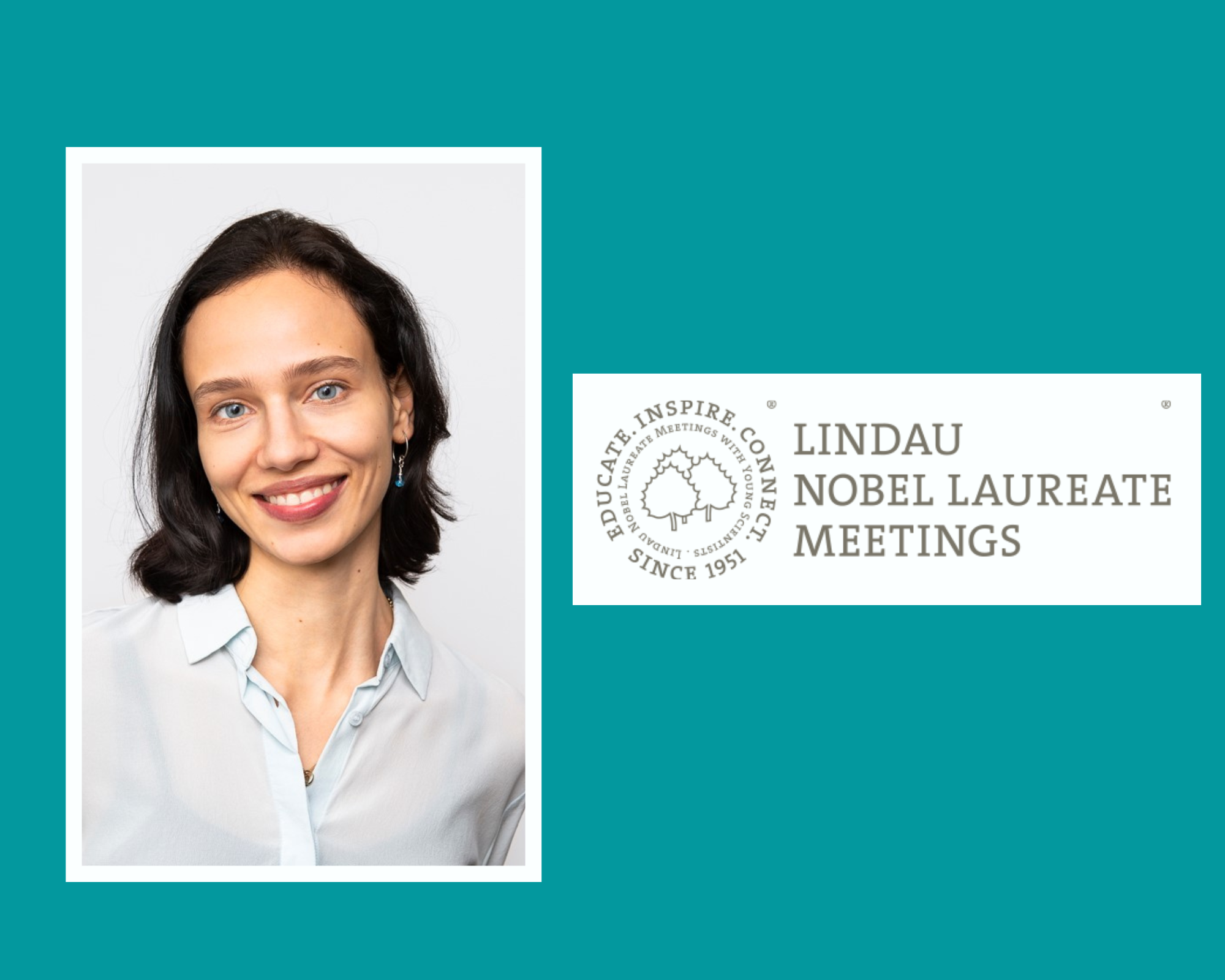
Sophia Rein - Selected to attend the 72nd Lindau Nobel Laureate Meeting
The Lindau Nobel Laureate Meetings – established in 1951 – provide a globally recognized forum for exchange between Nobel Laureates and young scientists. They inspire scientific generations and build sustainable networks of young scientists around the world.
You can learn more about the Lindau Nobel Laureate Meetings on their website here. Congrats to Sophia!
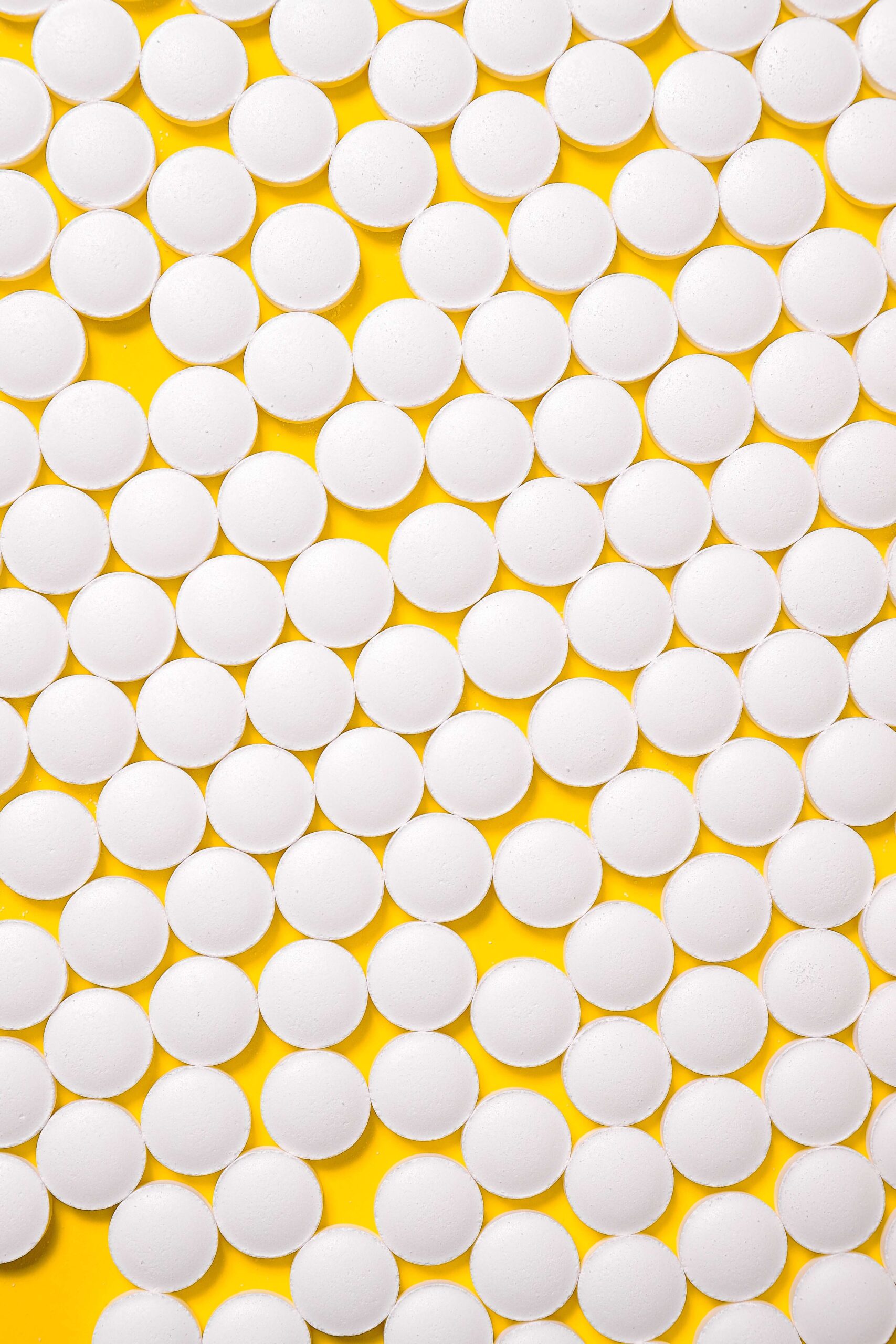
New Study: Estimating the per-protocol effect of lithium on suicidality in a randomized trial of individuals with depression or bipolar disorder
Because most randomized trials looking at the effect of lithium on the risk of suicidality (attempts, deaths, hospitalizations) have high rates of non-adherence, it is possible that a beneficial effect of lithium may have been diluted by the non-adherence and thus undetected by the intention-to-treat analysis. They reanalyzed the data from the CSP590 trial, a recent randomized trial conducted in the VA, to estimate the per-protocol effect of lithium use on recurrent suicidality among patients with affective disorders.
You can read the abstract and study here.
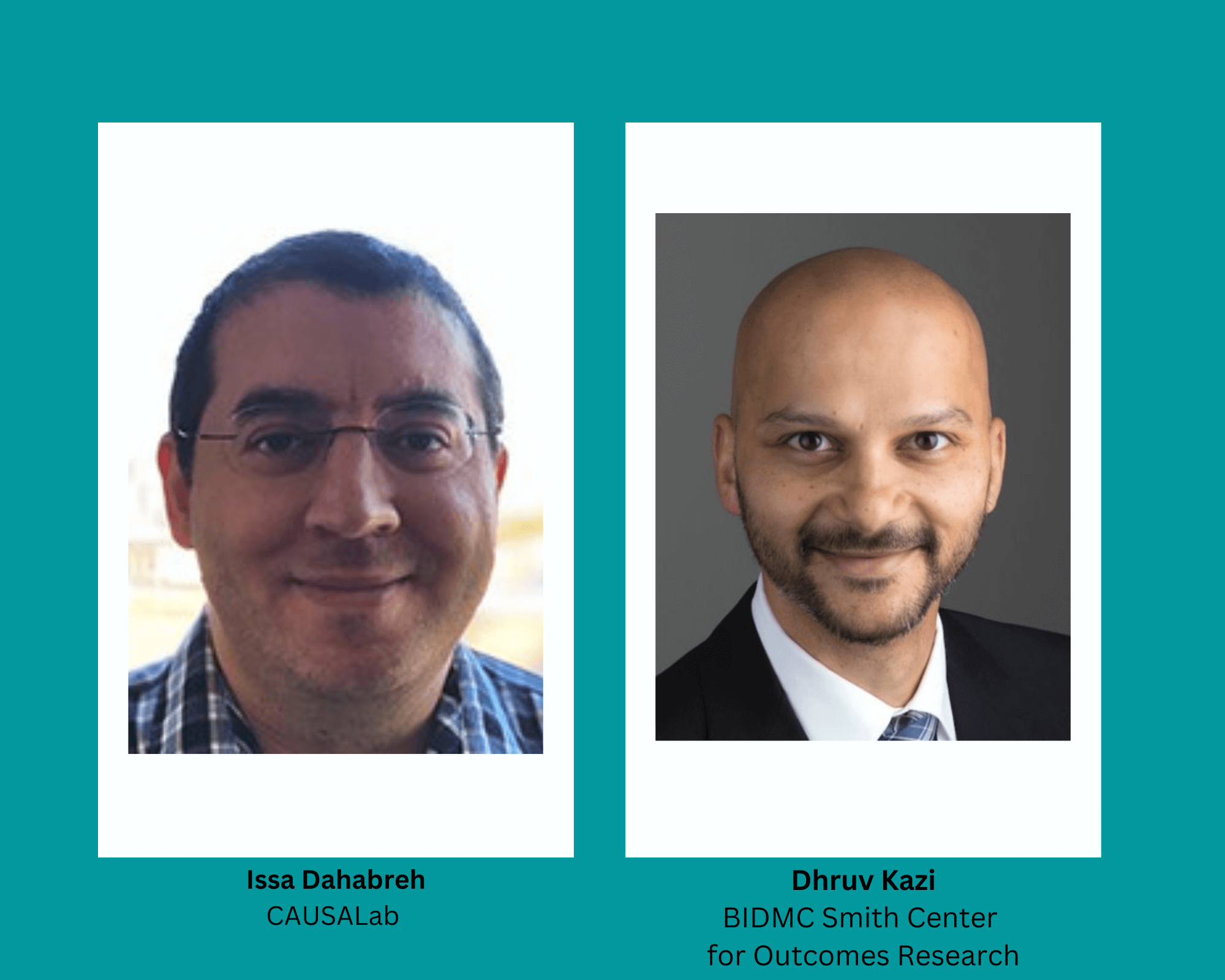
Editorial - Toward Personalizing Care: Assessing Heterogeneity of Treatment Effects in Randomized Trials
Dahabreh and Kazi highlighted in the abstract of their editorial, “Toward Personalizing Care: Assessing Heterogeneity of Treatment Effects in Randomized Trials” the following:
Clinicians know that individual patients may respond differently to a given treatment and that the overall treatment effect reported in a randomized trial of the treatment may not be directly applicable to all patients in clinical practice.1 Determining the treatment effect for an individual patient involves a comparison of the outcome when that patient is exposed to the treatment vs the outcome of the same patient exposed to a control treatment at the same time, a comparison impossible to make in conventional parallel-group trial designs. A practical alternative is to examine heterogeneity of (variation in) treatment effects across groups of patients, categorized by baseline demographic or clinical characteristics, such as age or risk factors for the outcome.2
To view the editorial piece, check it out on JAMA Network here.
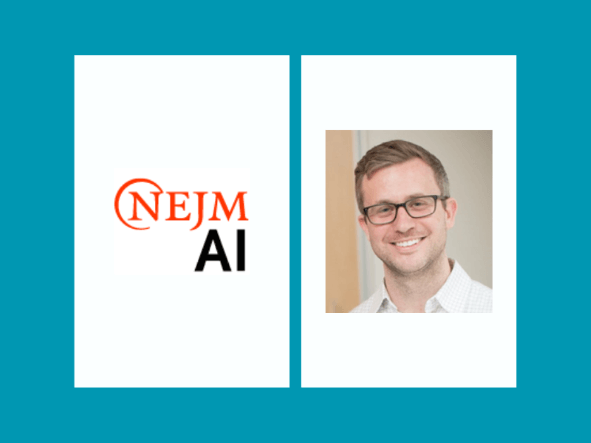
Andrew Beam - Founding Editor of New Journal, NEJM AI
NEJM will be published on a monthly basis online. Additionally, you can catch new monthly podcast of NEJM AI Ground Rounds Podcast and sign up for a twice-monthly newsletter to stay up to date on NEJM AI news.
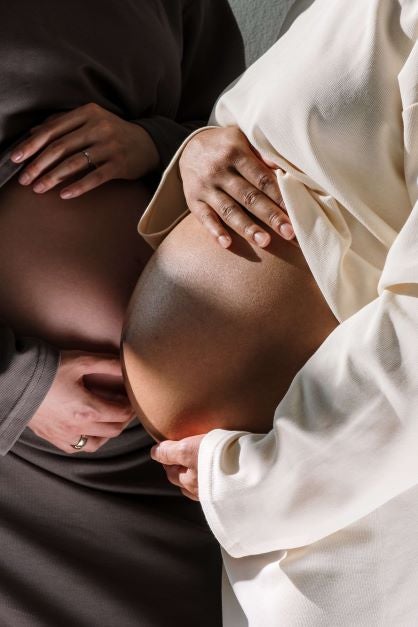
New Study: Emulating a Target Trial of Interventions Initiated During Pregnancy with Healthcare Databases: The Example of COVID-19 Vaccination
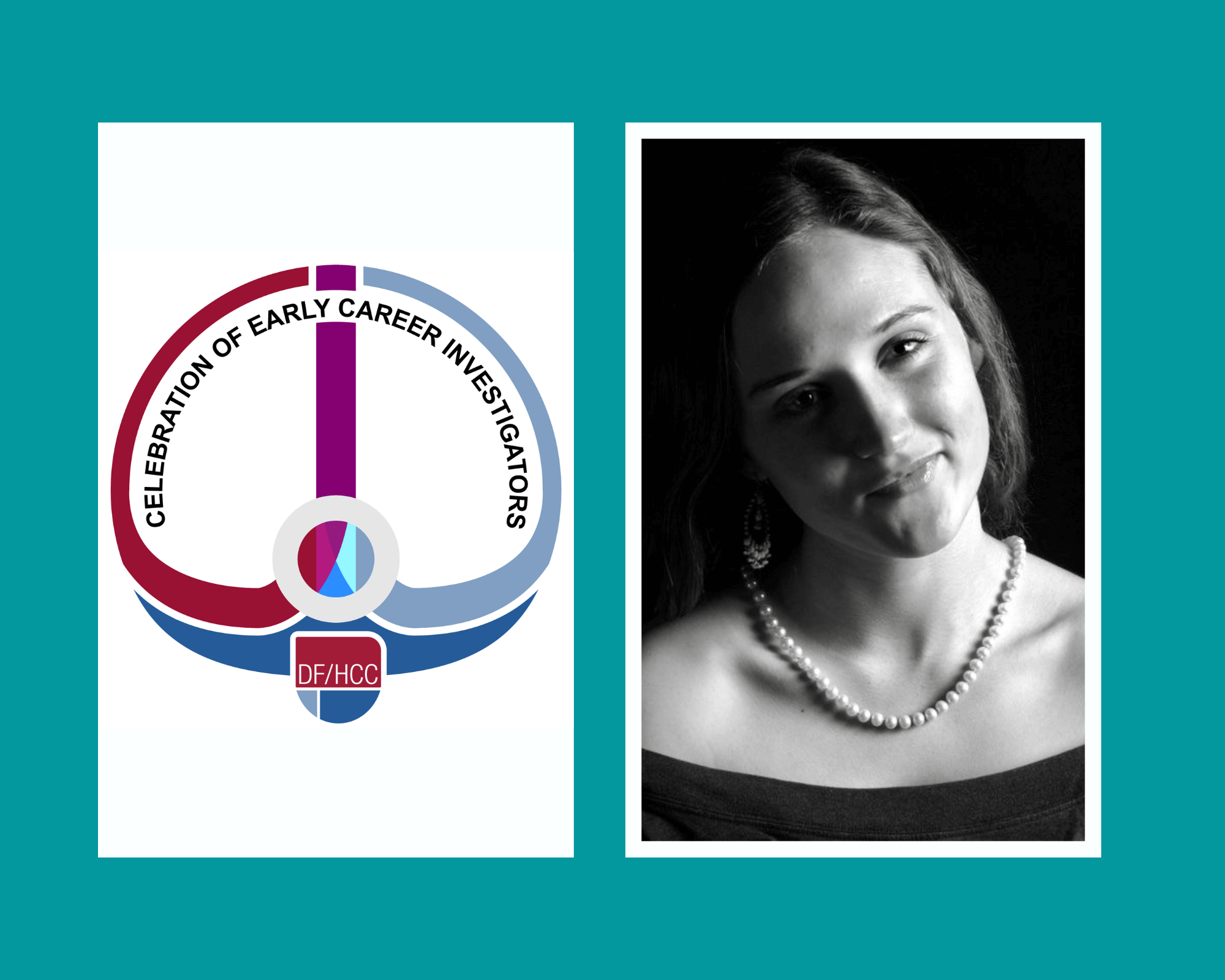
Emma McGee - Presenting at Dana-Harber/Harvard Cancer Center Symposium
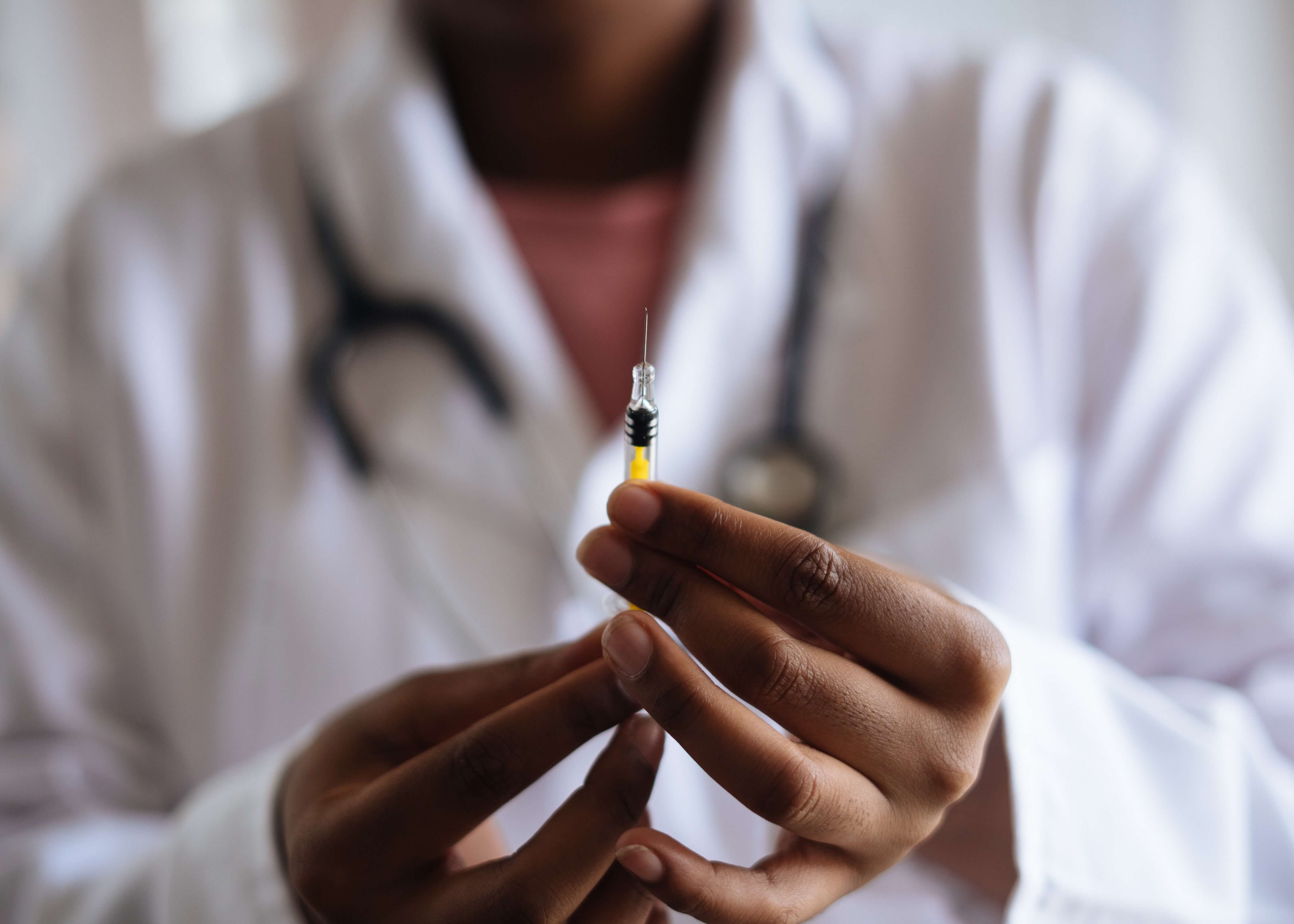
NEW STUDY: Comparative Effectiveness of Third Doses of mRNA-based COVID-19 Vaccines in US Veterans
CAUSALab researcher Barbra Dickerman was first co-author of this study along with Hanna Gerlovin of the Massachusetts Veterans Epidemiology Research and Information Center, VA Boston.
This study is published in the journal Nature Microbiology and can be found here.
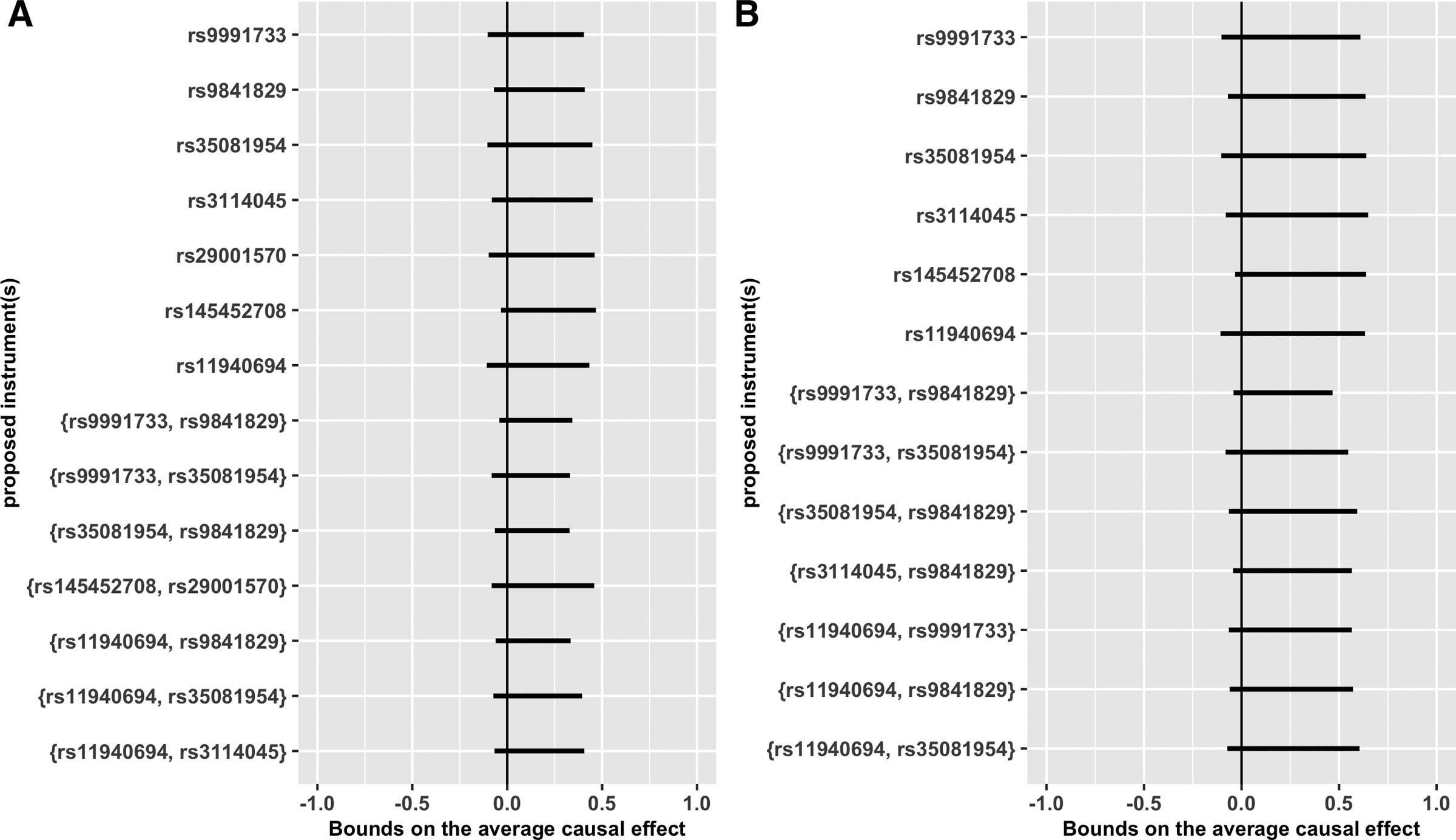
NEW STUDY: Partial Identification of the Average Causal Effect in Multiple Study Populations: The Challenge of Combining Mendelian Randomization Studies
This research was part of the HARVEST collaboration, supported by the Research Council of Norway (#229624).
You can read more about their study and results in the Journal of Epidemiology here.
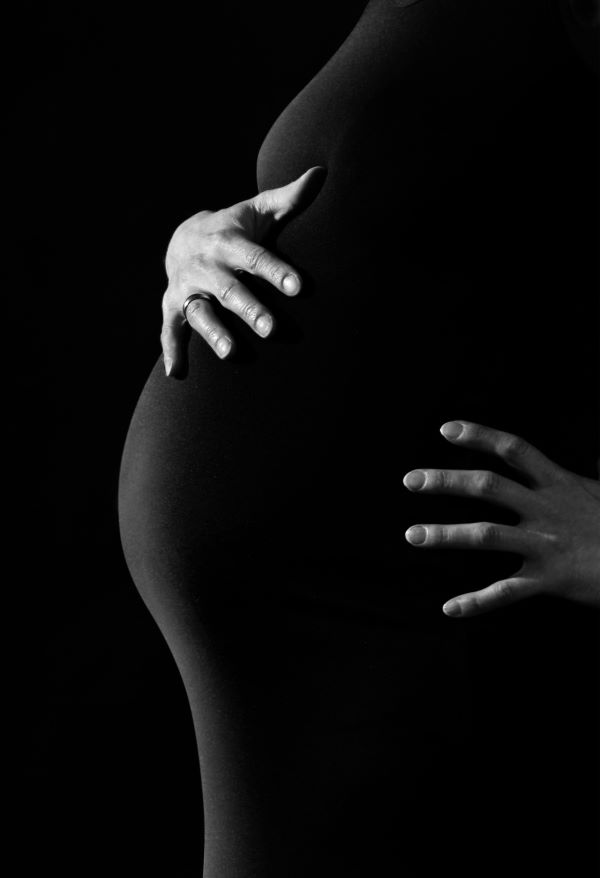
CAUSALab Research Cited as Evidence in FDA Recommendation
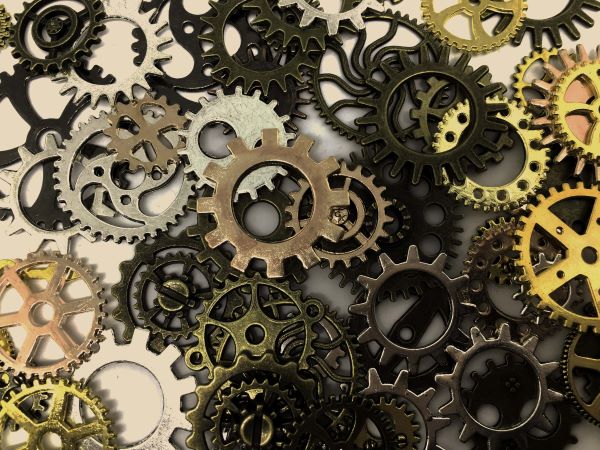
New Study: Deep Learning Methods for Proximal Inference via Maximum Moment Restriction
The No Unmeasured Confounding Assumption is widely used to identify causal effects in observational studies. Recent work on proximal inference has provided alternative identification results that succeed even in the presence of unobserved confounders, provided that one has measured a sufficiently rich set of proxy variables, satisfying specific structural conditions. However, proximal inference requires solving an ill-posed integral equation. Previous approaches have used a variety of machine learning techniques to estimate a solution to this integral equation, commonly referred to as the bridge function. However, prior work has often been limited by relying on pre-specified kernel functions, which are not data adaptive and struggle to scale to large datasets. In this work, we introduce a flexible and scalable method based on a deep neural network to estimate causal effects in the presence of unmeasured confounding using proximal inference. Our method achieves state of the art performance on two well-established proximal inference benchmarks. Finally, we provide theoretical consistency guarantees for our method.
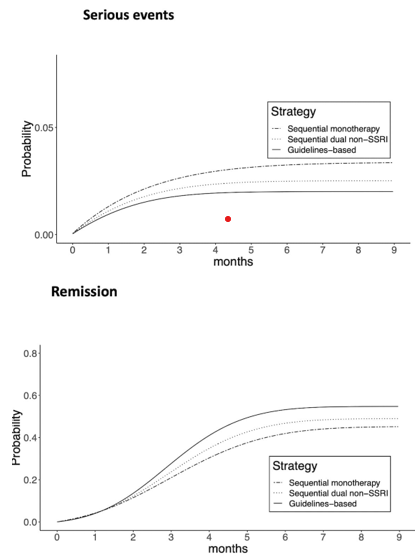
New Study: Emulating a target trial of dynamic treatment strategies for major depressive disorder using data from the STAR*D randomized trial
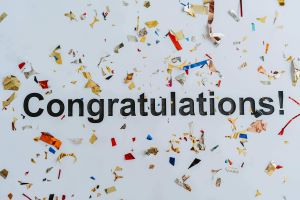
CAUSALab Researchers Win AJE and SER 2021 Articles of the Year
A list of all 2021 Articles of the Year will be released online and will appear in the American Journal of Epidemiology. Published dates forthcoming.
Thank you to the American Journal of Epidemiology and the Society for Epidemiologic Research for this recognition.
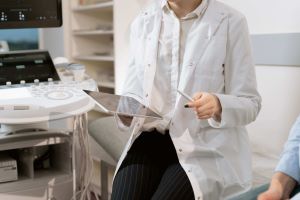
New Study: Effect of Colonoscopy Screenings on Risks of Colorectal Cancer and Related Death
The trial included 84,585 participants who were randomized to either receive an invitation to undergo a single colonoscopy screening or receive no invitation. Risk of colorectal cancer at 10 years was 18% lower among those who received an invitation (10-year risk of 0.98%) compared to those in the usual-care group (10-year risk of 1.20%). These findings are important for informing the relative benefits and harms of population-level colonoscopy screening programs and provide evidence for decision makers when prioritizing resources for comprehensive cancer control.
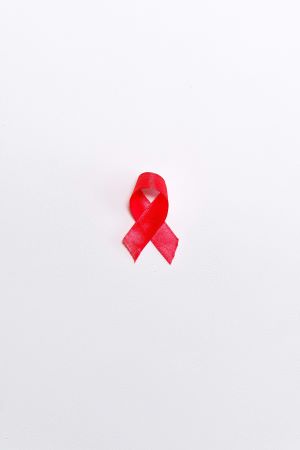
New Study: Tenofovir Disoproxil Fumarate and COVID-19 Outcomes in Men with HIV
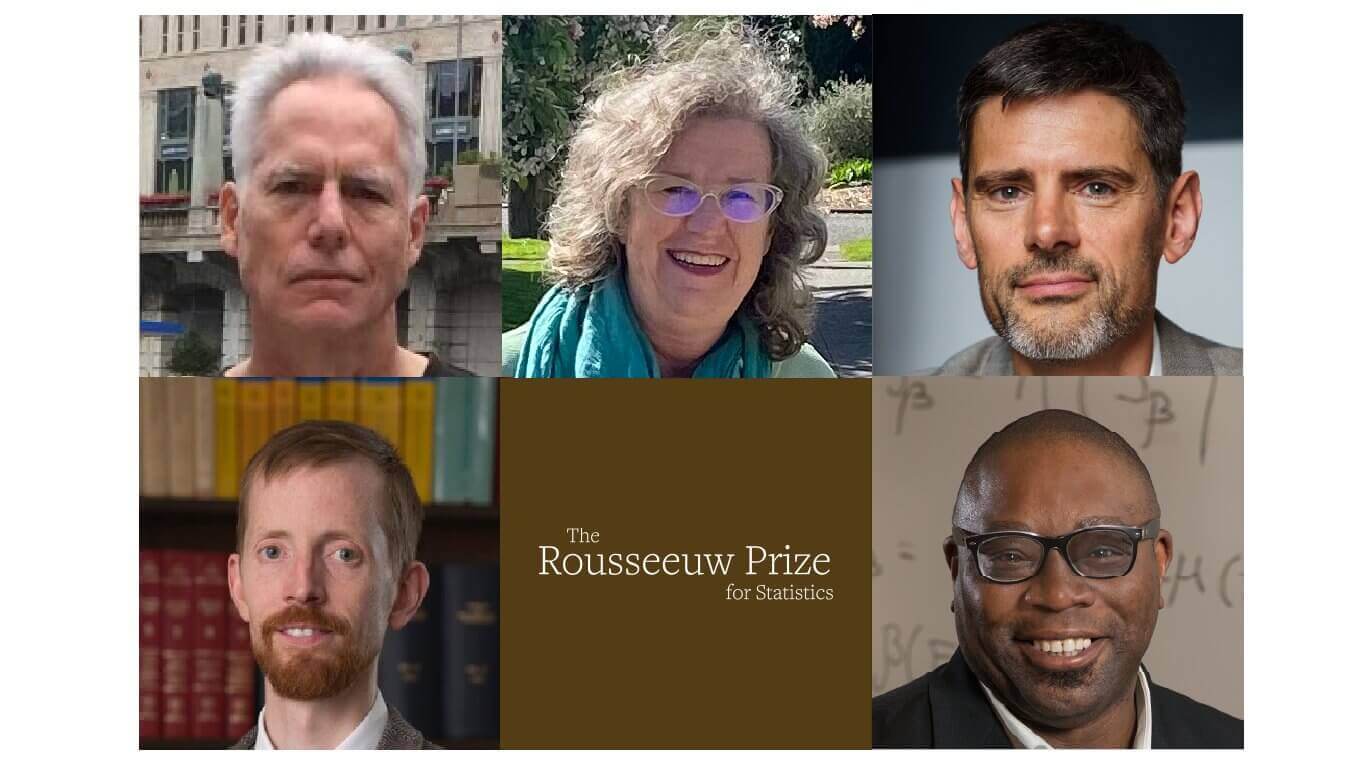
Jamie Robins & Miguel Hernan: Winners of the 2022 Rousseeuw Prize for Statistics
The King Baudouin Foundation has honored these laureates with the 2022 Rousseeuw Prize for Statistics for their work which has “completely transformed the way in which statisticians, epidemiologists, and others infer the effects of interventions, treatments, and exposures to potentially harmful substances. It has greatly improved the overall reliability of causal analysis in medicine and public health, with great benefit to society.”
As reported in KBF’s press release,
Half the prize amount will go to James Robins of Harvard University and half will be shared by Miguel Hernán (Harvard University), Thomas Richardson (University of Washington), Andrea Rotnitzky (Universidad Torcuato di Tella, Argentina) and Eric Tchetgen Tchetgen (University of Pennsylvania). The latter four laureates were either trained or deeply influenced by Robins. They remain his principal collaborators to this day.
The prize will be awarded at a ceremony taking place at University of Leuven, Belgium on Wednesday, October 12, 2022.
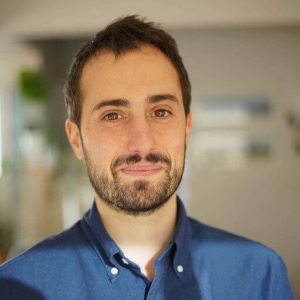
Alejandro Szmulewicz: Winner of the 2022 Young Investigator Grant by Brain and Behavior Research Foundation
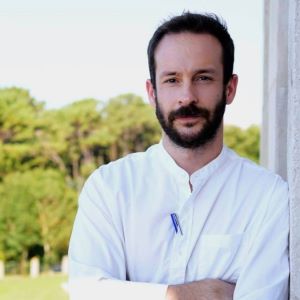
Gonzalo Martinez-Ales - Winner of the 2022 Young Investigator Grant by Brain and Behavior Research Foundation
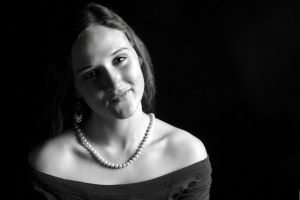